Amy Howard, Silei Zhu
Wellcome Centre for Integrative Neuroimaging (FMRIB Centre), University of Oxford
The BigMac dataset is an open access resource combining in vivo MRI, extensive postmortem MRI and multi-contrast microscopy for multimodal characterisation of a single whole macaque brain. The data is highly complementary to BigBrain facilitating complementary analyses across scales, datasets and species. Crucially, the BigMac MRI and microscopy data have been carefully co-registered together to facilitate quantitative multimodal analyses and data-fusion approaches which more heavily rely on multiple modalities being acquired in the same subject. This session will start with an introductory talk where I will outline the acquisition and curation of the BigMac data, alongside some example applications from the lab. The latter part of the session will feature hands-on interactive notebooks where participants can explore the BigMac data and curated outputs, as well as running microscopy-informed “hybrid” tractography and performing MRI-microscopy comparisons.
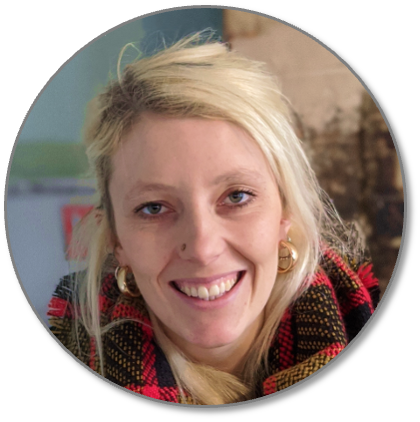
Amy Howard is a Postdoctoral Researcher at the Wellcome Centre for Integrative Neuroimaging (FMRIB Centre) at the University of Oxford, where she also completed her PhD. Her research focuses on combining quantitative MRI with microscopy to both better understand the origins of MRI signals and develop multi-scale, “data-fusion” methods to drive computational modelling of the brain microstructure.
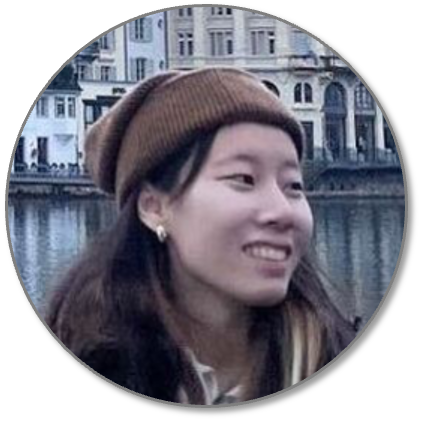
Silei Zhu is a PhD student at the Wellcome Centre for Integrative Neuroimaging (FMRIB Centre) at the University of Oxford. She completed her undergraduate study at Zhejiang University, China. Her research focuses on mapping brain connectome with integrative methods of combining MRI and microscopy. She developed open accessible tools using tractography, data fusion and deep learning for multi-scale and cross-species mapping of brain connectivity.